Data Storytelling for the Environmental Movement
360-401-DW 01
Course Description
N.B.: This course was developed by the Faculty of Political Science for the Environmental Studies profile.
We have lots of data, and we’ve got lots of problems to solve. This course explores how we can find and read climate related data to understand what needs to change, and then vulgarize what we’ve learned from the data for the general public.
To this end, students will learn how to read, work with, analyze, and argue with data, using tools that are focused, guided, inviting and expandable. This is not a lecture-based course: it is built on a large number of workshops that show students how to use technological tools to make things that help us think.
INTRODUCTION: THE CLIMATE STRIKE MOVEMENT
First, students are brought up to speed on the latest climate movement news and its global demands.
- Understanding the school strike for the planet. Guided viewing of David Suzuki’s Rebellion.
- WORKSHOP: collecting current climate strike demands from movement actors worldwide (assignment).
MODULE 1: FINDING AND TELLING STORIES WITH DATA
This module takes an in-depth look at what we mean when we talk about ‘data’. Then we examine how new technologies have turned data from something precious, to ubiquitous. We look at how and why data gets organized into datasets, and the emerging baseline standards for academic peer review of datasets. From there, we look at the inherent subjectivities in data, and we begin to examine how data can be used to tell a story.
Note on PowerPoints: in order to see the script associated with the slides, you need to open them on the PowerPoint app or PowerPoint for the web. (Once open, click on View and select ‘notes’).
What is data? (slides), The dataset? (slides)
- WORKSHOP: the analog spreadsheet (instructions)
What is the peer review process for datasets? (slides)
General peer review (assignment)
- WORKSHOP: write a blog post on the class blog about a data presentation you saw recently (assignment).
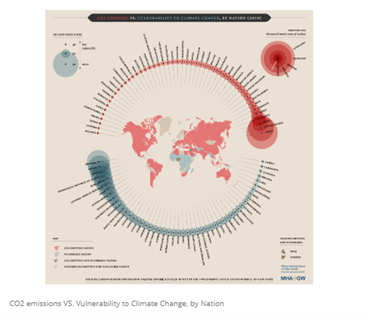
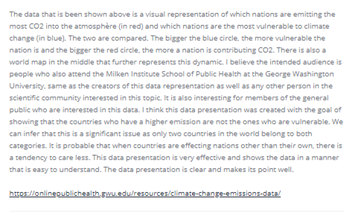
MODULE 2: ETHICAL PROBLEMS
This module begins by looking at how data can be used to influence others to act against their own interests. It searches out the most egregious examples and tells the story about what happened.
Then it switches course, to discuss how people are working to make data serve the interests of even the most marginalized members of society. Students will learn about current regulation, innovation and best practice for the effective use of data.
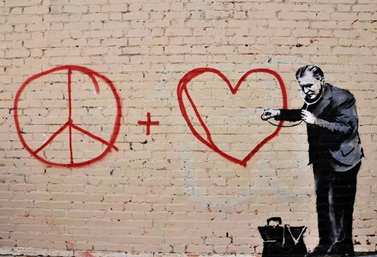
- Create an activity log of all the types of data you create during the course of one day (assignment).
- WORKSHOP: data log pair and share (instructions)
- Guided viewing of ‘The Great Hack’(questions)
- Reading comprehension (assignment)
Joel Gurin. 2014. Open Governments, Open Data: A New Lever for Transparency, Citizen Engagement, and Economic Growth. SAIS Review of International Affairs 34, 1 (2014), 71–82.
Michael B. Gurstein. 2011. Open data: Empowering the empowered or effective data use for everyone? First Monday 16, 2 (January 2011)
MODULE 3: GETTING AND CLEANING DATA
This module explores how getting data isn’t only about finding it, it’s about making it. In this spirit, we look at data scraping, and some of the requirements for proper data storage with structured and unstructured data, relational and non-relational databases. We then examine one of the most important topics in data analytics: data cleaning. Data cleaning is the bulk of the work.
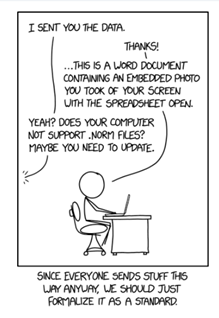
Where do we find data? (slides)
- WORKSHOP: Finding data for your questions (assignment)
Scraping data (slides), data storage strategies (slides), cleaning data: consistency and completeness (clip), cleaning data: usability and metadata (slides),
- WORKSHOP: Tidying data (instructions)
Bad spreadsheets are bad (slides), tools for cleaning (slides)
Brian Burghart. 2014. What I’ve Learned from Two Years Collecting Data on Police Killings. Gawker (August 2014).
Noah Veltman. 2013. Scraping the web. School of Data (November 2013).
Hadley Wickham. 2014. Tidy Data. Journal of Statistical Software 59, 10 (August 2014)
MODULE 4: ANALYSING DATA
This module endeavours to link back to Quantitative Methods and then beyond to help students understand some of the issues with interpreting results from a data set.
- TEAM LIGHTNING TALKS: (assignment)
Talk 1: Basic statistics recap
Talk 2: Exploratory data analysis
Talk 3: Common misunderstandings in statistics
Talk 4: the Null Hypothesis
Talk 5: Statistics and persuasion: the MAGIC criteria
Talk 6: Lying with statistics: P-hacking
Talk 7: Big data and inequity
Talk 8: Approaches to building data literacy
Talk 9: Small data
Talk 10: workshop – sketch a story
Talk 11: wordshop – deconstructing data visualizations
Gonick and Smith, Cartoon guide to statistics, William Morrow, 1993
Sameer Bhatnagar workshop
Abelson, Robert. “Making Claims with Statistics” from Statistics as Principled Argument Lawrence Eribaum Associates, 1995.
Aschwanden, Christie. “Science isn’t broken: it’s just a hell of a lot harder than we give it credit for.” FiveThirtyEight, Aug 19, 2015
“Can computers be racist? Big data, inequality, and discrimination”. Ford Foundation, 2015
Bhargava, Rahul and Catherine d’Ignazio. “Approaches to Building Big Data Literacy” Bloomberg data for good exchange conference, Sept. 2015.
Catherine D’Ignazio, Warren, Jeffrey, Blair, Don “Less is more: the role of small data for governance in the 21st century”.
Bhargava, Rahul “Sketch a Story” Data Culture Project, Databasic.io
Bhargava, Rahul “Deconstruct a Data Viz”, Data Culture Project, Databasic io
MODULE 5: TELLING STORIES WITH DATA
The great moment we’ve all been waiting for! We start by examining what makes persuasive data visualizations, then carefully, with the aid of worksheets and aide-memoires, students choose one from a number of small datasets, learn to ‘read’ the data with the assistance of wtfcsv and then they draw a data visualization, using traditional charts, creative charts, or mapping techniques.
They then learn to make dashboards to read larger datasets, after which they might use google studio or move straight to making data sculptures and more complex creative data stories. It feels very much like waiting to see if your birds take flight!
How not to (slides), How to (slides)
- WORKSHOP: Convince me (intro clip, instructions, image)
- WORKSHOP: Finding a Story (assignment, worksheets, datasets)
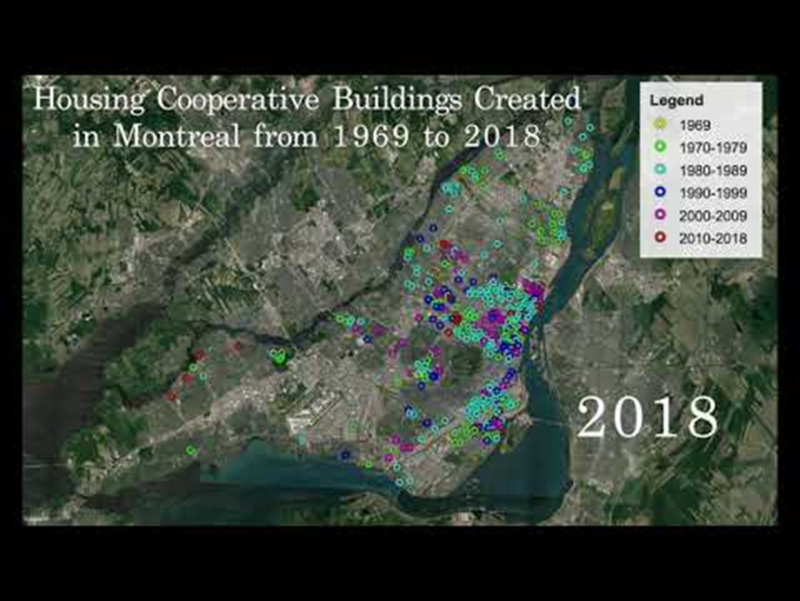
- WORKSHOP: Introduction to QUERY dashboards (assignment, workshop instructions – PART 1, workshop instructions – PART 2)
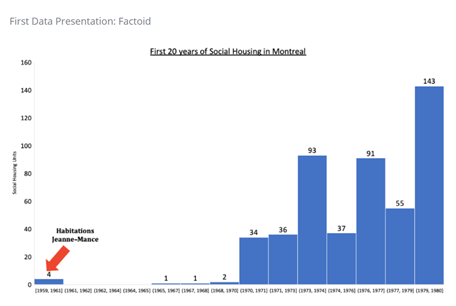
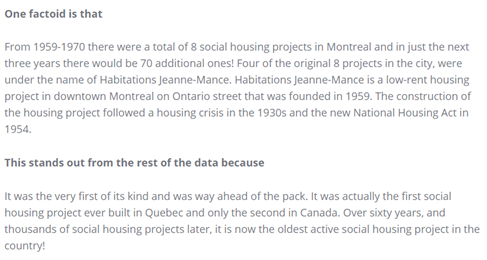
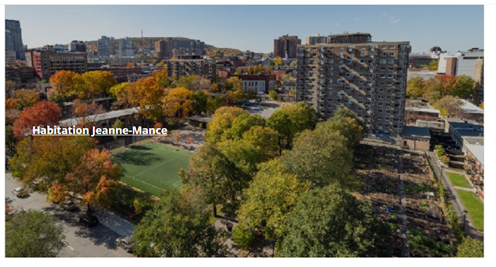
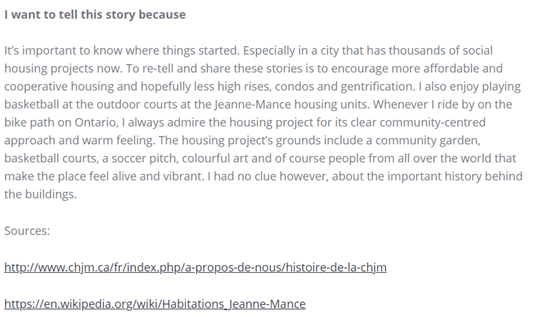