The Economics of AI
The rules of the game are always the same: compete, or else you lose your seat. This page will address the impacts of AI on the economy by attempting to answer several research questions:
- What are the similarities and differences between artificial intelligence and other technological advancements from an economics point of view?
- How can artificial intelligence contribute to environmental preservation without compromising
economic efficiency? - How can artificial intelligence enhance macroeconomic forecasting and reduce policy lags?
- Given its impact on the economy, how does artificial intelligence affect non-economic aspects of human life?
The content of this portfolio can be incorporated in all economics courses at the college level. The data analysis in the video below can be used in quantitative methods, and the part under “Beyond Economics” can be insightful in humanities and qualitative methods courses.
Labour Economics
AI is a general-purpose technology (GPT) which has been developed by governments and businesses to win a competitive edge over their rivals. Such technologies improve the economic conditions of employers, employees and households. However, this improvement is not evenly distributed across the sectors. GPTs increase the returns to those skills which are more compatible with the new means of productions. This itself is a signalling feature of the market mechanism, incentivizing labourers to specialize in areas which raise their productivity. It is exactly through this shift of balance that we achieve greater efficiency and higher standard of living—the most primary economic objective.
The following video provides a summary of how AI affects employment.
After meeting their primary needs, households tend to spend their marginal income predominantly on more labour-intensive products. This means a greater increase in the demand for services, compared to goods. We have observed this trend in the developed and developing countries over the 20th century. We can anticipate this to continue with AI, where the economy shifts from the goods sector to the services sector.
There is no value to employment in and of itself. Otherwise, we fall for the broken window fallacy, which is a major shortcoming of Keynesian economics. It is a fallacy to judge the economic impacts of an event by looking at only a single sector—such as the low-skilled workers—or at only its immediate effects in the short run.
Similarly, racial equity and gender representation are noble values which extend beyond the scope of economic objectives. Meanwhile, they can have heavy economic costs, which is often born by the relatively weaker sectors which were intended to be supported, especially when we push for quick substantial results before the natural course of economic forces can respond.
Improvement comes at some costs, and things are not all hunky-dory. However, it is neither desirable nor possible to resist technological advancement. Technology is a natural bottom-up flow that runs its course. The wisest strategy is to adapt ourselves to the new requirements and try to be on the leading front.
Environmental Economics
AI algorithms and machine learning (ML) can help economic agents—both consumers and producers—to overcome information asymmetry by lowering the cost of transparency. Real-time data on prices incentivize consumers to allocate their resources in the most efficient way. These algorithms have already been put in place in several industries such as energy, insurance, travel and tourism. When applied to route planning, variable toll roads, and autonomous vehicles, they can result in lower carbon footprint and less congestion. If similar algorithms are applied to garbage management, where households are charged according to the garbage they produce and the price of its disposal in their region, they can have further environmental benefits.
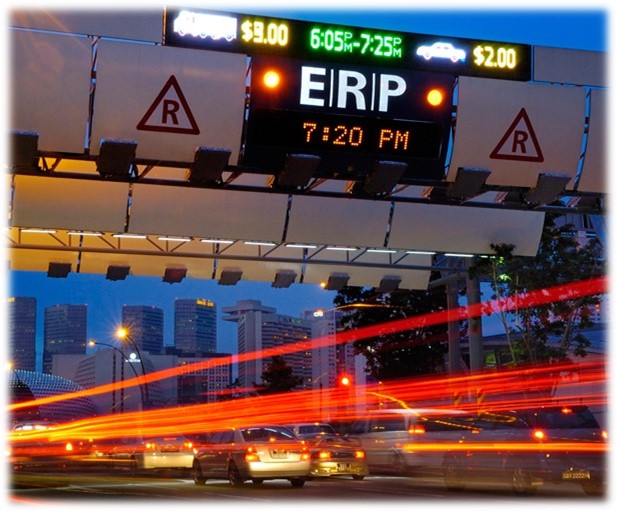
This is preferred to the current system of a flat price for energy, roads, and garbage disposal. It allows consumers to take more responsibility for their consumption patterns, and pay the cost of the negative externalities that they create. It is also more equitable.
Furthermore, machine learning and big data can make energy production more cost-effective environmentally in a number of ways:
- gauging the output of solar panels;
- forecasting the geological impacts of dams;
- assisting in the discovery and extraction of natural resources;
- predicting natural disasters, and thus avoiding major losses;
- enhanced farming methods with less use of pesticides and fertilizers.
Finally, prediction through AI processing uses less power. This itself is a direct environmental gain.
Macroeconomics
Economists have long abandoned the idea of fine-tuning the economy through fiscal and monetary mechanisms. However, gross-tuning remains popular, both in theory and practice. Nevertheless, the results have been far from perfect. Whether it was FDR’s New Deal in the 1930s or Obama’s Recovery Act of 2009, government programs have rather exacerbated business cycle fluctuations, mainly due to policy lags and the economic calculation problem.
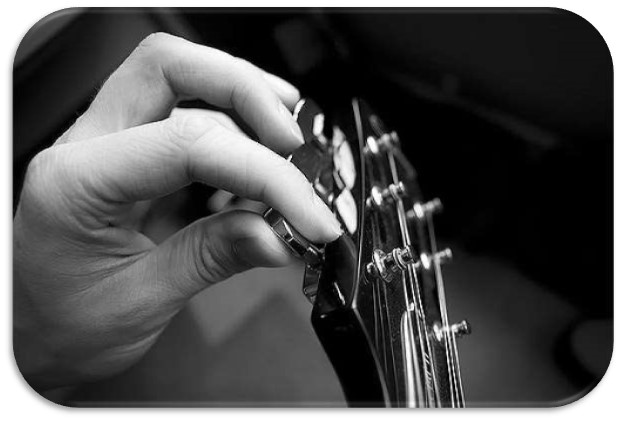
AI can prove helpful on this frontier in a number of ways, some of which overlap with microeconomics:
- Better job matches due to the use of AI will reduce unemployment, raise worker satisfaction, and result in higher productivity, which yields higher wages and thus a higher standard of living. This, after all, is the goal of macroeconomics.
- About 40% of the people in the world (over 3 billion) do not have internet access. The cost savings that come with AI will make internet spread out more, which will in turn enable more people to find suitable jobs. In addition to serving the relatively disadvantaged citizens of the world, this will also contribute to the world economy as whole. It can thus enhance efficiency, liberty and equity: three main socioeconomic goals.
- It has the capacity of filling gaps in data. Moreover, it can take non-quantitative data—such as court transcripts, email texts, social media connections, online reviews and calendars—and quantify them into new variables. Such data would be fitter for statistical analysis.
- It can save bureaucratic expenses due to savings on the cost of prediction, which should ideally translate into a lighter burden for the taxpayers.
- It can achieve the central bank’s inflation target by maintaining a steady and transparent monetary growth rate, without requiring extensive supervision.
- It can prevent financial bubbles through more accurate and efficient screening of loan applicants.
- It can replace government regulation, which is often susceptible to corruption and inefficiency. For instance, algorithms can predict hygiene levels based on customer reviews, which can serve as a substitute for costly FDA verifications.
- It can provide a breakthrough in public choice theory, as consumers get to actively participate in decisions which affect them through tweets. This gives voice to the consumers instead of bringing in policymakers and law enforcement.
- It can facilitate more efficient resource allocation in emergencies through systems like Enhanced 911 (E911). The additional data gleaned and processed by AI can help dispatchers prioritize their clients more effectively and equitably.
- A major source of happiness for people is a sense of trust in their fellow citizens, the economic system, and their government. The competitive nature of AI is likely to improve transparency and reduce the incentive to cheat, as observed in uberization.
- It can improve equality of opportunity and reduce generational poverty, as demonstrated by the Opportunity Insights project of Raj Chetty.
Beyond Economics
There is an overall consensus among economists that AI is not fundamentally different from other forms of technology. The economic analysis of AI offered above can contribute to a more balanced and comprehensive view of how this technology affects other areas of human life, such as liberty, morality and equity.
Outside the profession, the media often feeds on negativity and fear. That is understandable given their needs for publicity. What is unfortunate is to see academics hopping on this train of scaremongering, instilling fear into the students. There is no evidence to show any dramatic change in the economy due to the development of AI. This is not to idealize the situation. We are not in a utopia, nor heading towards one; but the world is not coming to an end either. Teachers in the academia should not turn into preachers in the media.
Freedom
Technological improvements save us time and free us from arduous tasks. While this is a valuable contribution, it is only the first step. That is what Erich Fromm calls “freedom from” as opposed to “freedom to” which is the next step. “Freedom from” is negative and external in nature: it is the absence of external constraints. That is why it is most visible. “Freedom to” is positive and internal: it is how we use our freedom actively, autonomously and responsibly, based on an inner sense of liberation and authenticity. This is less tangible. The two correspond to negative liberty and positive liberty, respectively, as coined later by Isaiah Berlin (1958). Fromm wrote in Escape from Freedom:
We neglect the role of the anonymous authorities like public opinion and “common sense,” which are so powerful because of our profound readiness to conform to the expectations everybody has about ourselves and our equally profound fear of being different. In other words, we are fascinated by the growth of freedom from powers outside ourselves and are blinded to the fact of inner restraints, compulsions, and fears, which tend to undermine the meaning of the victories freedom has won against its traditional enemies. We therefore are prone to think that the problem of freedom is exclusively that of gaining still more freedom of the kind we have gained in the course of modern history. (Fromm, 1969, pp. 125-126)
We escape from positive freedom because it comes with the unbearable burden of personal responsibility. The key to negative freedom is separation of power, because concentration of power corrupts. The key to positive freedom is inner strength and personal empowerment. AI gives us the first key since it is competitive and open access. It gives us “freedom from,” but then the question is what to do with it. Some forms of AI—such as the recommendation algorithms—may rather numb us to our inner freedom by isolating us in our bubble and giving us an exaggerated sense of confirmation. Thinkers and educators must take it upon themselves to foster this second stage of freedom through effective education. This, evidently, goes beyond the scope of economics. In short, we must appreciate the contribution of AI to human freedom in two ways: (1) by not stalling it through short-sighted impulses; (2) by specializing in the promotion of positive freedom. This is how humans and machines complement one another.
Idleness
Bertrand Russell has an insightful essay, “In Praise of Idleness.” He observes, as early as 1930s, that leisure is no longer the prerogative of the wealthy elite. “Modern technique has made it possible to diminish enormously the amount of labour required to secure the necessaries of life for everyone” (Russell, 2004, p. 5). He argues that in the industrialized countries, people can have a comfortable lifestyle working only four hours a day. The rest, he suggests, should be invested in what makes us more human. That would include a wide range of activities—depending on one’s background and conditions—which contribute to personal and professional development, such as art, literature, charity and community service.
This idleness ideally allows citizens to educate themselves, so that they may become more sensitive to their environment, may not fall for common fallacies, and may play a more active role on the political scene. Technology reduces the opportunity cost of leisure, which, if used wisely, can make us more responsible individuals, both in our own homes and to our neighbours. “Above all, there will be happiness and joy of life, instead of frayed nerves, weariness, and dyspepsia. The work exacted will be enough to make leisure delightful, but not enough to produce exhaustion” (p. 14).
We have seen this since the beginning of the 20th century, where leisure has become increasing more affordable to a greater proportion of the population. We can anticipate the same trend to continue with AI, resulting in a wide spreading of John William Godward’s Idleness:
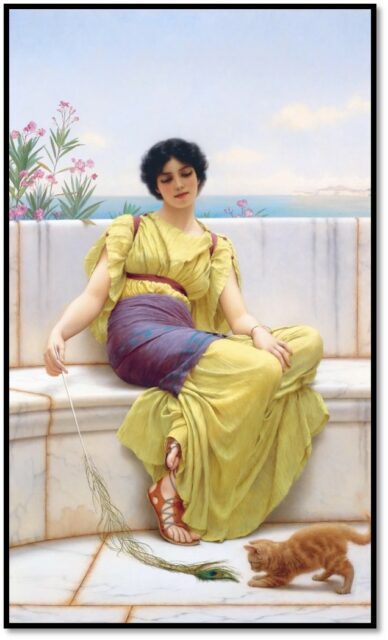
Ethical Dilemmas
The development of driverless cars is sometimes criticized for the challenges they pose on moral judgments and relative evaluations of human lives. This itself is a clear example of specialization. With technology taking care of the mechanics of driving, human minds are now free to address more fundamental questions. In other words, while some claim that AI is demoralizing and dehumanizing, we can already see that it is in fact awakening us to ethical issues which have been neglected for long. The fact that we are now asking and exploring these questions indicates a shift of focus in how we invest our resources, most notably human intelligence. This reorientation can be a stepping-stone toward the evolution of consciousness, as described by Sri Aurobindo.
More generally speaking, most of human history has been a struggle for survival, with an inevitable clash of interests and various forms of violence. It is only since the 19th century that we have gradually distanced ourselves from subsistence farming, thanks to rapid technological innovations. The satisfaction of our primary needs has allowed us to turn our attention toward higher needs and aim at promoting human rights, equality and peace. AI has the potential of fostering religious tolerance and also prompting us to face our inherent existential abyss. The complementarity of technology and ethics, among other aspects of human development, is expressed most eloquently by David Hume in his essay, “Of Refinement in the Arts”:
The same age, which produces great philosophers and politicians, renowned generals and poets, usually abounds with skilful weavers and ship carpenters. We cannot reasonably expect, that a piece of woollen cloth will be wrought to perfection in a nation, which is ignorant of astronomy, or where ethics are neglected. (Hume, 1994, p. 107)
Corporate Power
Like other forms of general-purpose technology (GPT), AI results in a more productive labour force. In the case of AI, the productivity gains accrue primarily to the consumers and employees, not to capital owners or purveyors, because of the of the technology.
Some thinkers—from within and without the discipline—have expressed concerns about companies such as Google, Apple and Microsoft gaining monopoly powers through AI. This is unwarranted because GPTs are not patented, and can be backward engineered easily. Hence, first-mover advantages are unlikely to be sustained, and AI is unlikely to be a huge money-maker for these companies in the long run. It is true that the data can be restricted, but the question to ask is: how scarce is that? Moreover, as AI technologies develop, they become less and less data-dependent. The marginal value of data is subject to the law of diminishing returns. In short, as long as the government does not cripple the industry through licence requirements, freedom of entry and exit will dissipate the market power of any single agent.
Equity
The employment-to-population ratio increases significantly in industries which use more technology. This positive labour-augmenting effect is even greater for women, which implies an advancement toward more equitable representation of women in high-tech jobs. Meanwhile, the wage premiums are also greater for college graduates than for non-college graduates. This means that while the gender wage gap is decreasing, an inner split may be widening between college and non-college graduate female workers.
It must be noted that the greatest concerns around the development of AI are based on ethical, not technical, grounds. Machine learning (ML) is an algorithmic processing of data. If the available data is biased, it will inevitably lead to biased results by AI. This can exacerbate the current level of bias in a given field.
Although this is concerning, it also carries a silver lining, because it serves to bring hidden biases to the forefront. In addition, ML has the capacity to predict unconscious biases of human decision makers, as demonstrated in recent studies. Therefore, its role can be compared to the function of wine according to Rumi:
It’s not in every head that wine would create
Evil, but its role is just to escalate.
The wicked becomes more wicked by drinking;
But the wise would become greater in thinking.
While it is imperative to acknowledge the limitations of AI—whether economic or ethical, short-term or long-term—it is also imperative for academics and educators not to put on the garb of propagandists, and not to lose their touch with empirical evidence. Hafez, also using the wine metaphor, drives this message home:
You listed the vices of wine, well done;
How about its virtues? They’re also a ton.
Do not conceal the fact of the matter
To appeal to a public who’re going to scatter.
References and Recommended Readings
The first and foremost resource to consult is The Economics of AI, including a rich bank of conferences and papers. The recordings of the NBER annual conference at Toronto are also available for years 2017, 2018 and 2019.
Here is a list of sources consulted in the creation of this portfolio, including the above video.
Abdulov, R. (2020). Artificial intelligence as an important factor of sustainable and crisis-free economic growth. Procedia Computer Science, 169, 468-472.
Acemoglu, D., & Restrepo, P. (2018). Artificial intelligence, automation and work (No. w24196). National Bureau of Economic Research.
Acemoglu, D., & Restrepo, P. (2019). Automation and new tasks: How technology displaces and reinstates labor. Journal of Economic Perspectives, 33(2), 3-30.
Acemoglu, D., & Restrepo, P. (2020). Robots and jobs: Evidence from US labor markets. Journal of Political Economy, 128(6), 2188-2244.
Agrawal, A., Gans, J., & Goldfarb, A. (2018). Prediction machines : The simple economics of artificial intelligence. Boston, Massachusetts: Harvard Business Review Press.
Autor, D., & Salomons, A. (2017). Does productivity growth threaten employment?. In ECB Forum on Central Banking, Sintra, Portugal (pp. 26-28).
Autor, D., & Salomons, A. (2018). Is automation labor-displacing? Productivity growth, employment, and the labor share (No. w24871). National Bureau of Economic Research.
Becker, G. S., & Posner, R. A. (2009). Uncommon sense: economic insights, from marriage to terrorism. University of Chicago Press.
Bhargava, R. (2018). The algorithms aren’t biased, we are. MIT Media Lab. https://medium.com/mit-media-lab/the-algorithms-arent-biased-we-are-a691f5f6f6f2
Brynjolfsson, E., Rock, D., & Syverson, C. (2018). Artificial intelligence and the modern productivity paradox: A clash of expectations and statistics. In The economics of artificial intelligence: An agenda (pp. 23-57). University of Chicago Press.
Dillender, M., Forsythe, E. C. (2019). Computerization of white collar jobs. Upjohn Institute Working Paper 19-310. Kalamazoo, MI: W. E. Upjohn Institute for Employment Research. https://doi.org/10.17848/wp19-310
Fromm, E. (1969). Escape from freedom. New York: Avon Books.
Furman, J. (2016, July). Is this time different? The opportunities and challenges of artificial intelligence. In K. Crawford & M. Whittaker (Cochairs), AI Now: The social and economic implications of artificial intelligence technologies in the near term. Symposium conducted at the meeting of the AI Now Institute, New York, NY.
Garver, R. (2017). Most powerful women in finance: No. 10, JPMorgan Chase’s Joyce Chang. American Banker. https://www.americanbanker.com/news/most-powerful-women-in-finance-2017-no-10-joyce-chang
Hume, D. (1994). Political Essays (K. Haakonssen, Ed.). Cambridge: Cambridge University Press.
King, K. (2019). Using Artificial Intelligence in Marketing: How to harness AI and maintain the competitive edge. Kogan Page Publishers.
Langlotz, C. P., Allen, B., Erickson, B. J., Kalpathy-Cramer, J., Bigelow, K., Cook, T. S., … & Kandarpa, K. (2019). A roadmap for foundational research on artificial intelligence in medical imaging: from the 2018 NIH/RSNA/ACR/The Academy Workshop. Radiology, 291(3), 781-791.
Layard, R. (2011). Happiness: lessons from a new science. New York: Penguin Press.
Melnychenko, O. (2019). Application of artificial intelligence in control systems of economic activity. Virtual Economics, 2(3), 30-40.
Mokyr, J. (2018). The past and the future of innovation: Some lessons from economic history. Explorations in Economic History, 69, 13-26.
Mokyr, J., Vickers, C., & Ziebarth, N. L. (2015). The history of technological anxiety and the future of economic growth: Is this time different?. Journal of economic perspectives, 29(3), 31-50.
Rowe, E. (2019). Capitalism without capital: The intangible economy of education reform. Discourse: Studies in the Cultural Politics of Education, 40(2), 271-279.
Russell, B. (2004). In praise of idleness and other essays. London; New York: Routledge.
Statistics Canada. Table 14-10-0020-01 Unemployment rate, participation rate and employment rate by educational attainment, annual. https://www150.statcan.gc.ca/t1/tbl1/en/tv.action?pid=1410002001#tables
Statistics Canada. Table 14-10-0023-01 Labour force characteristics by industry, annual (x 1,000). https://doi.org/10.25318/1410002301-eng
Statistics Canada. Table 14-10-0204-01 Average weekly earnings by industry, annual. https://doi.org/10.25318/1410020401-eng
Statistics Canada. Table 14-10-0327-01 Labour force characteristics by sex and detailed age group, annual. https://doi.org/10.25318/1410032701-eng
Statistics Canada. Table 36-10-0208-01 Multifactor productivity, value-added, capital input and labour input in the aggregate business sector and major sub-sectors, by industry. https://doi.org/10.25318/3610020801-eng
Susskind, D. (2020). A world without work: Technology, automation and how we should respond. Penguin UK.